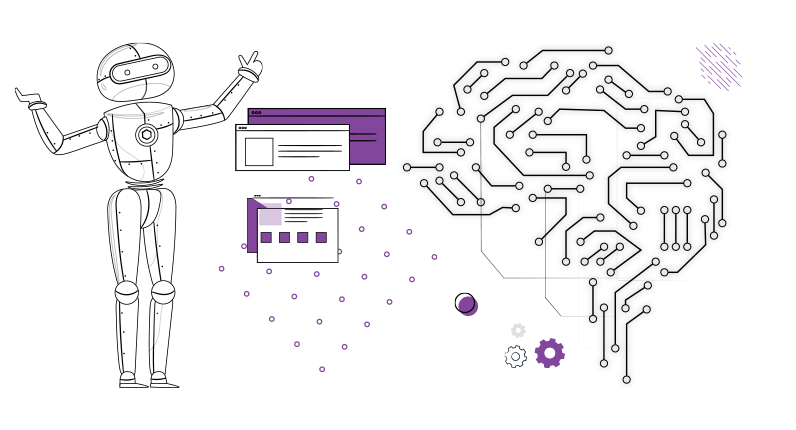
What is Responsible AI?
Responsible AI is the practice of designing, developing, and deploying AI with good intention to empower employees and businesses, and fairly impact customers and society – allowing companies to engender trust and scale AI with confidence. Source
While the opportunities of Responsible AI are for real, primarily to augment human beings, with bottom-line gains that directly impact the operational efficiencies and improved user experience, there is plenty of concerns on the trust factor, accuracy, privacy and data security factors.
Unlike heuristic approaches, any machine learning based applications mature with age, usage, feedback and constant fine turning – just like how human learning works!
Here are simple practices that we follow in our AI products and also spend adequate time to recommend to our customers towards improving the usage, adoption and success of their AI applications.
Continuous Learning
Just like humans, machines cannot be the know-it-all on day 1 and they need to be trained and learn continuously. Issues will happen and no machine learning model is perfect on day 1. Learn from user feedback, error scenarios and the changing environment in which they operate.
Trust in the AI systems is key to improve All our AI solutions include training workflows to continuously retrain the machine learning models, especially around Speech, Language for the Voice and NLP based solutions we build.
Transparency
As much as machines learn and produce results to aid humans, it is equally imperative on the part of the machines (primarily the application software) not to be like a black box. The AI output generation mechanisms should be simple and easy to understand for the users, backed by evidence of how the system produced the output.
Feedback loop
Follow a metrics driven approach to gather user feedback on any possible error scenarios after every AI based output. ‘Do you feel this is incorrect’, ‘Did I help you get the right answer?’, ‘Do you find this information really useful?’.
When in doubt, handle ambiguity with prompts like ‘Did you mean this?’ and let your application act on those feedback and continuously mature the accuracy of the results.
Monitor, measure and grow.
Build sufficient probes in place within your application to collect real-time click stream data, user feedback, responses and errors after every deployment. Include necessary KPIs to provide transparency on these measures that helps improve the application.